Leaders are ignoring the dangers of ‘confidently incorrect’ AI: Why it’s a massive problem
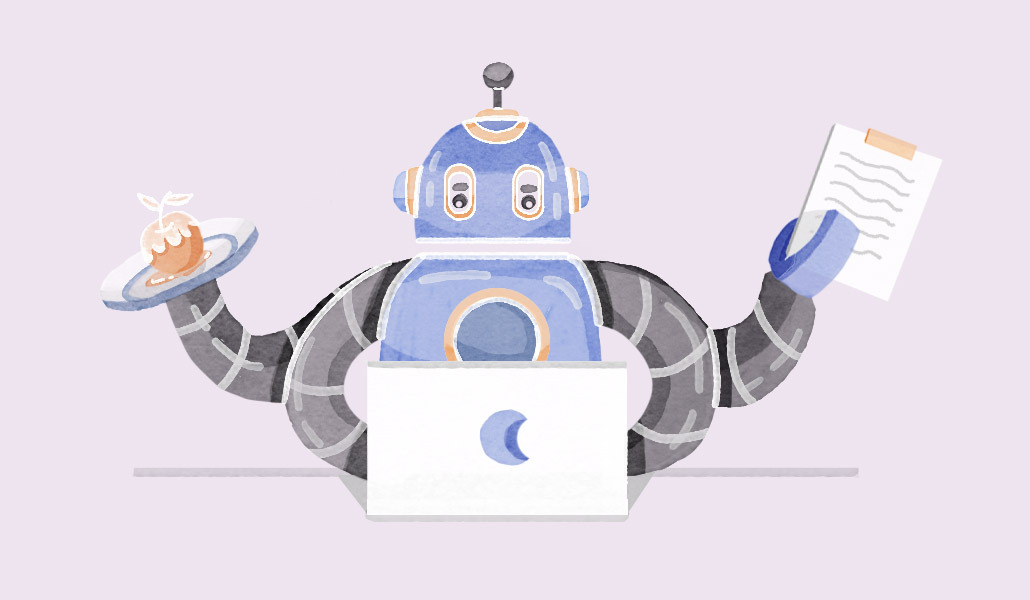
Why don’t scientists trust atoms? Because they make everything up.
When Greg Brockman, president and co-founder of OpenAI, demonstrated the possibilities of GPT-4 – Generative Pre-trained Transformer 4, the fourth-generation autoregressive language model that uses deep learning to produce human-like text – upon launch on Mar. 14, he tasked it to create a website from a notebook sketch.
Brockman prompted GPT-4, on which ChatGPT is built, to select a “really funny joke” to entice would-be viewers to click for the answer. It chose the above gag. Presumably, the irony wasn’t purposeful. Because the issues of “trust” and “making things up” remain massive, despite the incredible yet entrancing capabilities of generative artificial intelligence.
Many business leaders are spellbound, stated futurist David Shrier, professor of practice (AI and innovation) at Imperial College Business School in London. And it was easy to understand why if the technology could build websites, invent games, create pioneering drugs, and pass legal exams – all in mere seconds.
Those impressive feats are making it more challenging for leaders to be clear-eyed, said Shrier, who has written books on nascent technologies. In the race to embrace ChatGPT, companies, and individual users, are “blindly ignoring the dangers of confidently incorrect AI.” As a result, he warned that significant risks are emerging as companies rapidly race to re-orient themselves around ChatGPT without being aware of – or ignoring – the numerous pitfalls.
While ChatGPT is a large-scale language model, having been fed over 300 billion words by developer OpenAI, it is prone to so-called “hallucinations.” This was the term used by Google researchers in 2018 to explain how neural machine translations were “susceptible to producing highly pathological translations that are completely untethered from the source material.”
Essentially, as these models were – and still are – prone to spiraling off course, or becoming delusional, much like the human brain, it would be wrong not to fact-check what is produced.
AI: inherently stupid
“If these hallucinations are not caught before being published, then at best you are providing your audience with false information, and at worst opening your business up to significant reputational damage,” warned Greg Bortkiewicz, digital marketing consultant at Magenta Associates, a U.K. integrated communications consultancy.
Assurances from OpenAI that GPT-4 would be “safer and more aligned … and 40% more likely to produce factual responses,” according to a blog post that accompanied the release, should be taken with a fistful of sodium. Even co-founder Sam Altman admitted the latest version was “still flawed, still limited.” And he added that “it still seems more impressive on first use than it does after you spend more time with it.”
Bortkiewicz’s take on GPT-4 was: “It can hallucinate answers and produce inappropriate content, and it still doesn’t know about events after Sept. 2021.” He advised organizations to use it similarly to its predecessor: namely, as a “support tool rather than a content production tool – and with plenty of human oversight.”
Indeed, U.K.-based technologist James Bridle agreed in an essay – published by The Guardian, coincidentally, a couple of days after GPT-4 was launched – that AI “is inherently stupid.” He wrote: “It has read most of the internet, and it knows what human language is supposed to sound like, but it has no relation to reality whatsoever.”
As such, Bridle urged leaders to take an ambivalent approach to ChatGPT and AI in general. He added that believing it was “knowledgeable or meaningful [was] actively dangerous. It risks poisoning the well of collective thought, and of our ability to think at all.”
Put another way, relying on AI to work its magic could make organizations cut corners, and if stakeholders found out, this might be brand-damaging.
Sending the wrong message
For instance, in late February, officials at Vanderbilt University, Tennessee, were forced to issue a hasty apology after using ChatGPT to write a consoling email, signed by two administrators (but with the small print revealing the actual author), to students following a mass shooting at Michigan State University. Bridle noted how the original message “felt both morally wrong and somehow false or uncanny to many.” But, he added: “It seems there are many areas of life where the intercession of machines requires some deeper thought.”
Moreover, training data deployed in real-world conditions will cause the AI to misbehave. Victor Botev, CTO and co-founder at Iris.ai, a Norway-headquartered start-up focused on researching and developing AI technologies, asked: “ChatGPT has been trained with millions upon millions of scraped text samples, but how many internet forums or blog posts contain obscure scientific terminology? And of those, how many even use them correctly?” Therefore, he argued that funding this area of intense study for AI researchers was critical. So too, would be ensuring appropriate checks and balances were in place.
How worrying, then, that Microsoft – an organization that in January announced a new “multiyear, multibillion-dollar investment [in OpenAI] to accelerate AI breakthroughs” – recently laid off an ethical AI team.
Botev stressed the importance of establishing and strengthening guardrails, upgrading the robustness of data structures, and shifting the mindset and data from “quantity to quality.” He said large language models offered “endless possibilities, but their turn in the spotlight exposes unsolved questions: factual accuracy, knowledge validation, and faithfulness to an underlying message.”
The Bulgarian national pointed out that while Microsoft and other large corporations had “the resources and technological heft” to tackle some of the issues regarding general search results, “this won’t work for everybody.”
Botev added: “To solve these issues for niche domains and business applications requires substantial investment. Otherwise, these models are unusable. Ultimately, to build trust in AI’s decision-making process, we must prioritize explainability and transparency.”